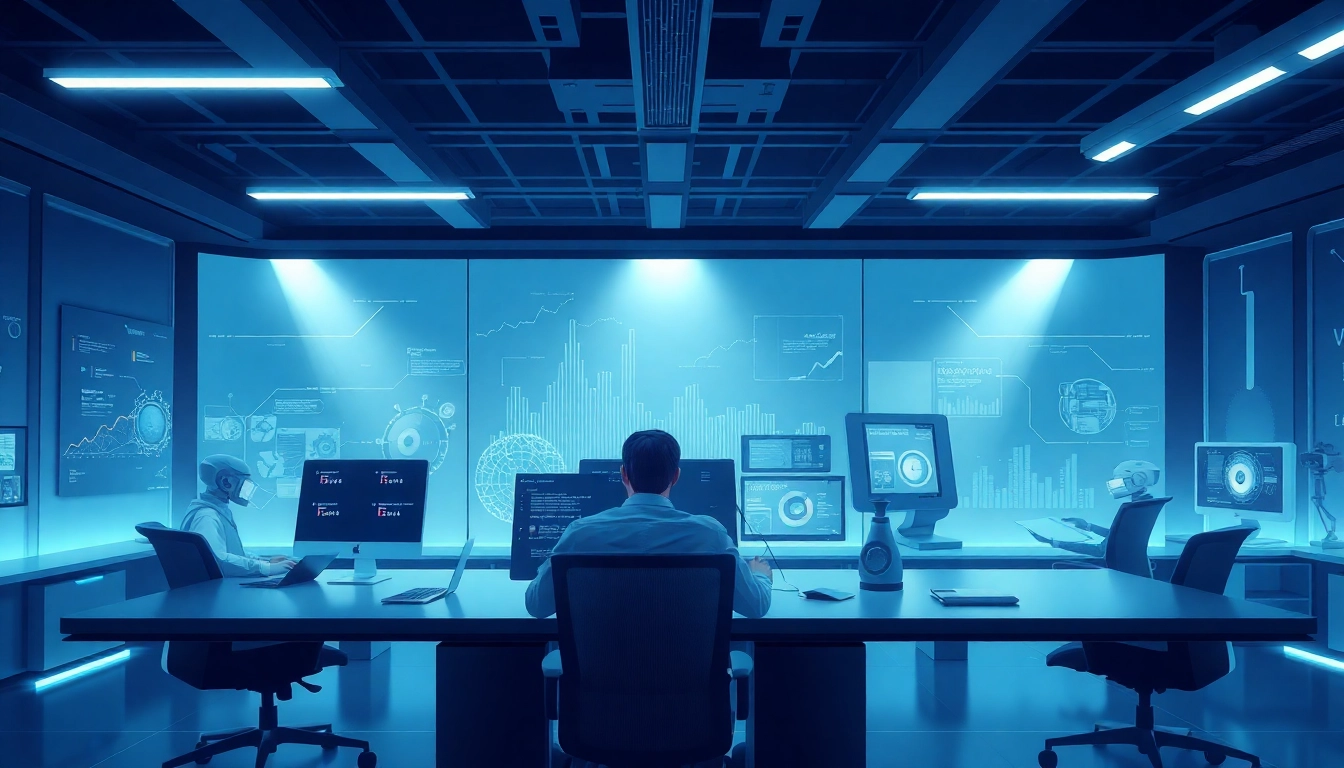
Understanding Agentic AI
In an era marked by rapid advancements in artificial intelligence, Agentic AI emerges as a pivotal concept reshaping the landscape of autonomous systems. Unlike traditional AI, which often requires extensive human oversight, agentic AI possesses the capability to make decisions and act independently, thus empowering it to achieve complex goals with limited human intervention. This article delves into the foundational principles, applications, challenges, and future trends associated with agentic AI, aimed at providing readers with a comprehensive understanding of this transformative technology.
Definition and Core Principles
Agentic AI refers to intelligent systems designed to operate autonomously, using sophisticated reasoning and iterative planning to navigate complex, multi-step problems. At its core, agentic AI integrates concepts from various disciplines, including machine learning, cognitive science, and systems theory, to create models that can learn from their environment, adapt to new situations, and make informed decisions without continuous human input. This approach contrasts with generative AI, which primarily focuses on content creation driven by user prompts.
Key Characteristics of Agentic AI
The distinguishing attributes of agentic AI encompass several key characteristics:
- Autonomy: The defining feature of agentic AI is its ability to function independently. It can perceive its environment, make choices, and execute tasks based on its programming and past experiences.
- Adaptability: Agentic AI systems can adjust their strategies in response to changing circumstances, learning from outcomes to enhance future performance.
- Goal-directed behavior: These systems are designed to pursue specific objectives, employing optimal strategies to accomplish their tasks efficiently.
- Reasoning capabilities: Through advanced algorithms, agentic AI systems can perform complex reasoning, mimicking human-like decision-making processes.
Distinguishing Features from Other AI Types
It is essential to distinguish agentic AI from other forms of AI, particularly generative AI and traditional rule-based systems. Generative AI focuses on producing new content—texts, images, or sounds—while agentic AI is mission-oriented, resulting in active participation in problem-solving scenarios. Moreover, traditional AI systems often rely on predefined algorithms and human inputs to function, lacking the autonomy that characterizes agentic models. This autonomy allows agentic AI to operate in dynamic environments, making it particularly suited for complex applications, such as robotics, autonomous vehicles, and smart systems.
Applications of Agentic AI
As businesses and organizations strive for efficiency, scalability, and competitive advantage, the application of agentic AI is rapidly gaining traction across diverse sectors. The potential use cases are extensive and varied.
Automating Business Processes
One of the most significant applications of agentic AI lies in the automation of business processes. Organizations can deploy agentic AI to streamline operations, reduce operational costs, and enhance productivity. For instance, companies are leveraging automated decision-making systems in supply chain management to optimize inventory levels, forecast demand, and reduce wastage through real-time analysis and adaptive strategies. These systems utilize machine learning algorithms to process vast amounts of data, driving efficiency while limiting human oversight.
Enhancing Customer Experience
Enhancing customer experience is another key area where agentic AI demonstrates its transformative potential. By employing chatbots and virtual assistants powered by agentic AI, businesses can offer personalized customer interactions that respond dynamically to individual needs and preferences. These agents continuously learn from past interactions, enabling them to predict customer inquiries and provide tailored support. As a result, companies can significantly enhance engagement while reducing response times, leading to improved customer satisfaction and loyalty.
Utilizing Agentic AI in Supply Chain Management
In the realm of supply chain management, agentic AI optimizes logistics, planning, and execution processes by making real-time decisions based on fluctuating data inputs. For example, AI systems can autonomously allocate resources during supply chain disruptions, minimizing delays and operational inefficiencies. By utilizing predictive analytics, agentic AI forecasts supply chain trends and adjusts strategies accordingly, allowing organizations to maintain a competitive edge while fostering resilience against market uncertainties.
Challenges and Considerations
As with any technological evolution, the implementation of agentic AI comes with its share of challenges and ethical considerations that organizations must navigate.
Ethical Implications of Autonomous Decision-Making
The ethical implications of autonomous decision-making by AI systems raise significant questions regarding accountability, transparency, and bias. As agentic AI operates independently, establishing clear accountability for decisions made by these systems becomes imperative. Organizations must ensure that their AI implementations are transparent and that mechanisms are in place to audit and review decision-making processes. This is particularly critical in sensitive sectors, such as finance and healthcare, where erroneous AI decisions can lead to detrimental outcomes.
Technical Limitations of Current Systems
While agentic AI has made significant strides, technical limitations still hinder its full realization. The complexity of human environments requires AI systems to process a myriad of variables and contextual cues, which can be challenging for current algorithms. Issues related to scalability, data quality, and integration within existing systems remain prevalent. Addressing these challenges necessitates continuous enhancements in AI research and development, focusing on creating more robust frameworks capable of dealing with real-world complexities.
Addressing Public Perception and Trust
Public perception and trust in AI systems are crucial for successful implementation. Many individuals harbor fears about AI taking over jobs or making decisions without human oversight. To mitigate these concerns, organizations need to invest in effective communication strategies that emphasize the benefits of agentic AI in enhancing human capabilities rather than replacing them. Engaging stakeholders and providing education on AI’s role can foster a more positive perception and encourage broader acceptance.
Future Trends in Agentic AI
The evolution of agentic AI is poised for significant advancements, influencing various industries and societal norms. As technology continues to evolve, it’s essential to consider the future trends shaping the agentic AI landscape.
Emerging Technologies and Innovations
Emerging technologies such as quantum computing, advanced neural networks, and edge computing are expected to facilitate the development of more sophisticated agentic AI systems. These innovations will enhance the processing power, speed, and efficiency of AI systems, allowing them to solve complex problems more effectively. Additionally, advancements in natural language processing can enable more intuitive interactions between humans and AI agents, leading to more meaningful engagements.
Predictions for AI and Job Dynamics
As agentic AI systems become more prevalent, the dynamics of employment and job functions will shift significantly. Many roles may evolve rather than disappear, as AI takes over repetitive and mundane tasks, allowing human workers to focus on more strategic, creative, and human-centric activities. Industries will require workers to possess skills in collaboration with AI systems, creating a demand for training programs that equip employees with the necessary capabilities for this new era of work.
Agentic AI’s Role in Sustainable Development
Agentic AI holds great promise in driving sustainable development initiatives by optimizing resource use and promoting environmentally friendly practices. For instance, in agriculture, AI-driven solutions can improve crop yield predictions, enabling better resource allocation and management. Similarly, agentic AI can optimize energy use in smart cities, promoting sustainable living through enhanced environmental monitoring and resource management systems. By harnessing the potential of agentic AI, organizations can contribute to the achievement of sustainable development goals while maintaining economic viability.
Getting Started with Agentic AI
For organizations looking to implement agentic AI solutions, several critical steps must be taken to ensure effective deployment and continued efficacy.
Implementing Agentic AI Solutions
The path to implementing agentic AI solutions begins with identifying specific business challenges or opportunities where these systems can deliver value. This requires a comprehensive assessment of existing processes and clarity regarding the objectives to be achieved with AI. Organizations should consider collaborating with AI experts to curate tailored solutions that align with their unique requirements. A phased implementation approach can also facilitate smoother transitions, allowing organizations to gradually integrate AI capabilities and performance metrics effectively.
Measuring Performance and Success
Measuring the performance and success of agentic AI systems involves defining key performance indicators (KPIs) aligned with the objectives set during implementation. Organizations should track metrics related to efficiency, accuracy, cost savings, and customer satisfaction. Continuous monitoring and evaluation are essential to identify areas for improvement and optimize the AI’s effectiveness. Data-driven insights can guide future enhancements and strategic decisions, ensuring sustained success.
Continual Learning and Adaptation Strategies
The future success of agentic AI systems hinges on their ability to learn and adapt over time. Organizations should adopt a culture of continual learning, encouraging AI systems to refine their strategies based on new data and experiences. This can involve incorporating feedback loops, conducting regular audits, and sharing insights across teams. Furthermore, implementing frameworks that allow agentic AI to integrate real-time data can significantly enhance responsiveness and resilience in rapidly changing environments.